Predictive Analytics Harnessing Data for Future Insights
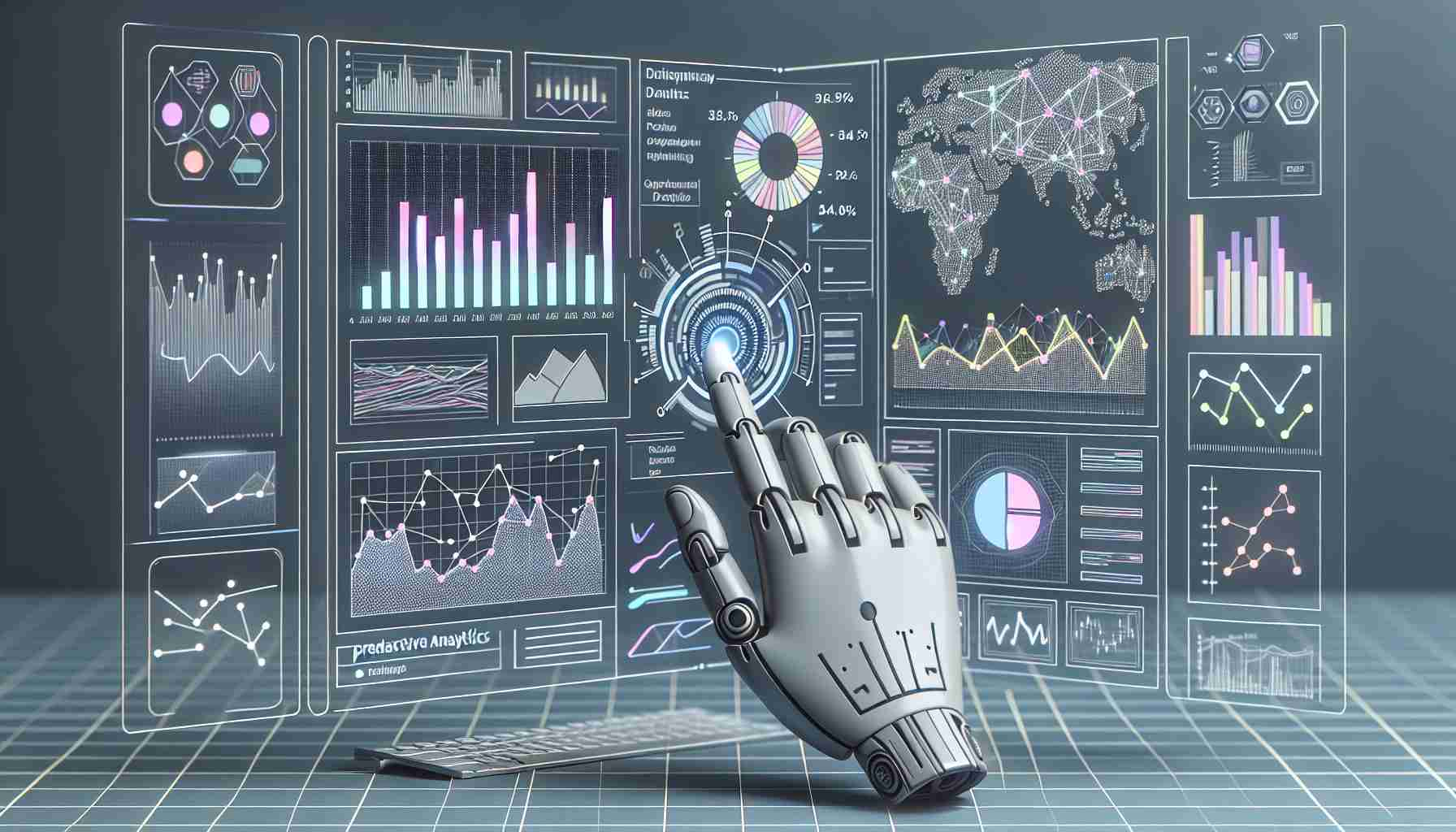
Predictive Analytics Briefly Summarized
- Predictive analytics involves the use of historical data to forecast future events and trends.
- It employs techniques such as data mining, statistics, data modeling, artificial intelligence, and machine learning.
- The goal is to identify patterns and insights that can inform decision-making and strategic planning.
- Predictive models are used across various industries, including healthcare, finance, marketing, and more.
- Benefits include improved decision-making, risk reduction, and enhanced ability to anticipate future demands or issues.
Predictive analytics represents a significant leap in the capacity of data to inform and guide strategic decisions. By analyzing historical data and identifying patterns, businesses and organizations can make more informed predictions about future events, behaviors, and trends. This powerful tool is reshaping industries by offering insights that were previously inaccessible, allowing for proactive rather than reactive strategies.
Introduction to Predictive Analytics
At its core, predictive analytics is about understanding the past to predict the future. It is a multidisciplinary field that combines various statistical techniques, machine learning algorithms, and data modeling practices to analyze current and historical facts to make predictions about future or otherwise unknown events.
Predictive analytics is the bridge between raw data and actionable insights. It turns large volumes of data into a strategic asset that can be used to make more informed decisions. By leveraging predictive analytics, organizations can identify risks and opportunities much earlier, which can lead to competitive advantages and improved outcomes.
The Process of Predictive Analytics
The predictive analytics process typically involves several key steps:
- Data Collection: Gathering large amounts of relevant data from various sources.
- Data Cleaning and Preparation: Ensuring the quality of data by cleaning and structuring it appropriately.
- Data Analysis: Using statistical techniques to explore and analyze the data.
- Model Building: Developing predictive models using machine learning algorithms.
- Validation and Testing: Assessing the model's performance using new data.
- Deployment: Implementing the model into operational systems.
- Monitoring and Maintenance: Continuously monitoring the model's performance and updating it as necessary.
Techniques and Tools in Predictive Analytics
Predictive analytics utilizes a variety of techniques and tools, including:
- Data Mining: Extracting patterns from large datasets.
- Statistical Analysis: Using statistical methods to interpret data and relationships.
- Machine Learning: Applying algorithms that improve automatically through experience.
- Predictive Modeling: Creating, testing, and validating a model to best predict the probability of an outcome.
Applications of Predictive Analytics
Predictive analytics has a wide range of applications across different sectors:
- Healthcare: Predicting patient outcomes, managing hospital resources, and identifying at-risk populations.
- Finance: Assessing credit risk, detecting fraudulent transactions, and algorithmic trading.
- Marketing: Personalizing customer experiences, optimizing campaigns, and managing customer relationships.
- Supply Chain: Forecasting demand, managing inventory, and optimizing logistics.
The Impact of Predictive Analytics
The impact of predictive analytics on business and society is profound. It enables organizations to:
- Make data-driven decisions that reduce risk and uncertainty.
- Anticipate customer needs and market changes.
- Optimize operations and increase efficiency.
- Innovate by identifying trends and opportunities.
Challenges and Considerations
While predictive analytics offers numerous benefits, it also presents challenges:
- Data Privacy: Ensuring the ethical use of data and protecting individual privacy.
- Data Quality: The accuracy of predictions is heavily dependent on the quality of the data used.
- Model Complexity: Complex models may be difficult to interpret and explain to stakeholders.
- Changing Environments: Models may become outdated as the underlying environment changes.
Conclusion
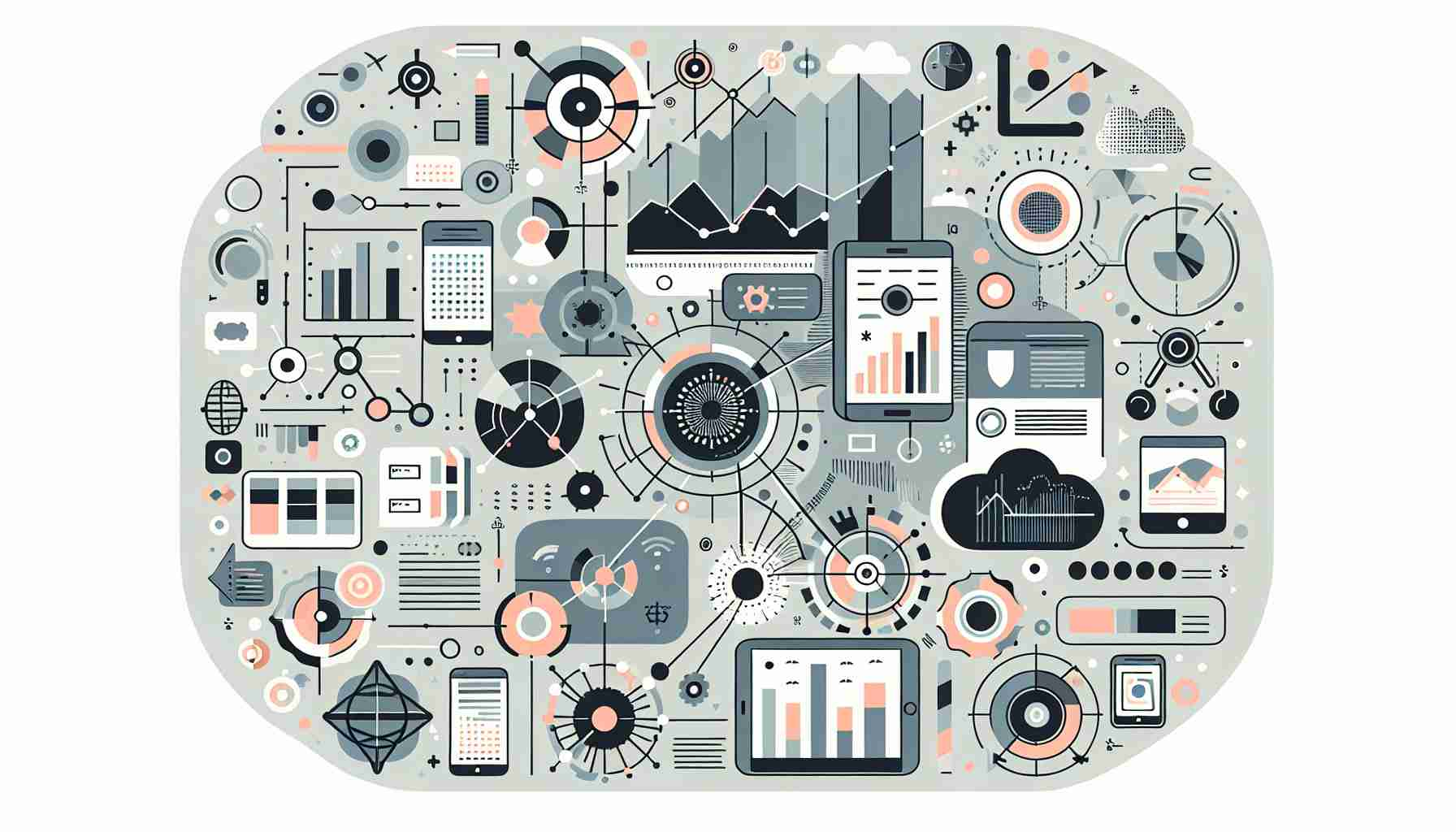
Predictive analytics is a transformative tool that allows organizations to look into the future and make more informed decisions. By understanding and applying the principles and techniques of predictive analytics, businesses can unlock the full potential of their data, gaining insights that drive success and innovation.
FAQs About Predictive Analytics
What is predictive analytics? Predictive analytics is the practice of using historical data, statistical algorithms, and machine learning techniques to predict future outcomes.
How does predictive analytics differ from other forms of analytics? Unlike descriptive analytics, which looks at past performance, or prescriptive analytics, which suggests actions, predictive analytics focuses on forecasting future probabilities and trends.
What industries can benefit from predictive analytics? Virtually all industries can benefit from predictive analytics, including healthcare, finance, marketing, retail, manufacturing, and more.
What are the key challenges in predictive analytics? Challenges include ensuring data privacy, maintaining data quality, managing complex models, and adapting to changing environments.
How accurate is predictive analytics? The accuracy of predictive analytics depends on the quality of the data, the robustness of the models, and the stability of the environment in which they are applied. It is not about predicting the future with certainty but about estimating probabilities and trends.
Sources
- What Is Predictive Analytics? 5 Examples | HBS Online
- What is Predictive Analytics? - IBM
- A Guide To Predictive Analytics - Tableau
- Predictive Analytics: Definition, Model Types, and Uses - Investopedia
- What Are the Benefits of Predictive Analytics in Healthcare?
- Predictive Analytics: What it is and why it matters - SAS
- Predictive analytics - Wikipedia
- What is Predictive Analytics? An Enterprise Guide - TechTarget
- What is predictive analytics? Transforming data into future insights