Analytics Maturity Model
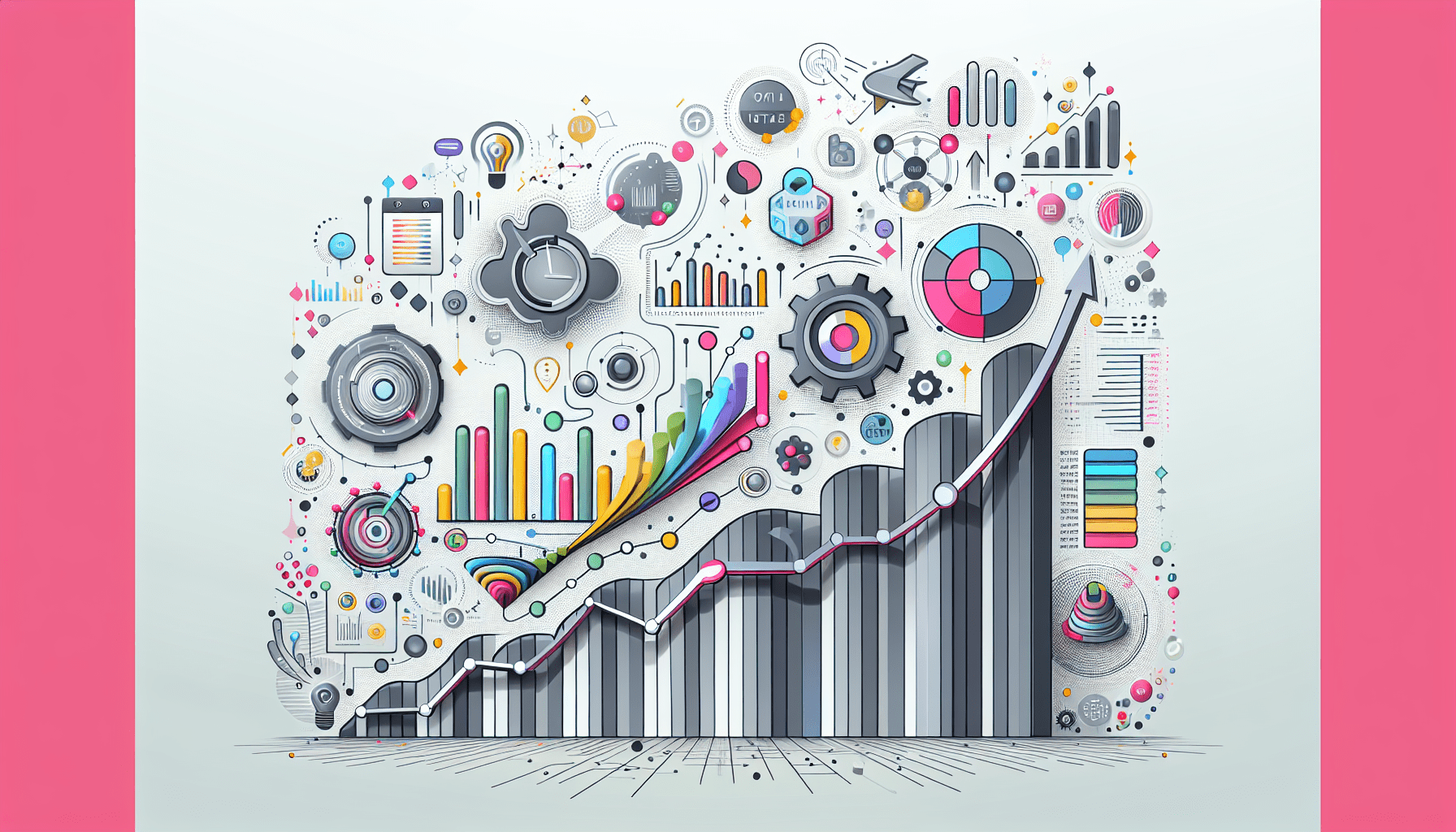
Briefly Summarized
- An Analytics Maturity Model (AMM) is a framework that outlines the stages of a company's analytics capabilities, from basic data management to advanced analytics.
- It serves as a roadmap for organizations to assess their current level of analytics proficiency and to plan the development of more sophisticated analytics practices.
- The model typically includes stages such as Descriptive, Diagnostic, Predictive, and Prescriptive analytics.
- AMMs help organizations to align their analytics strategies with business goals, optimize decision-making, and gain a competitive edge.
- The maturity of an organization's analytics can be a significant factor in its overall performance, efficiency, and innovation.
The concept of an Analytics Maturity Model (AMM) has become increasingly important as organizations strive to harness the power of data in their decision-making processes. In a data-driven world, the ability to effectively analyze and interpret data is not just a competitive advantage but a necessity for survival and growth. This article will delve into the intricacies of the Analytics Maturity Model, exploring its levels, technologies, applications, and its role in guiding organizations toward analytics excellence.
Introduction to Analytics Maturity Model
The Analytics Maturity Model is a structured framework that helps organizations identify their current analytics capabilities and provides a pathway for progression to more advanced levels of analytics. It is a strategic tool that enables businesses to measure and monitor their progress in the field of data analytics, ensuring that their investments in data and analytics are aligned with their overall business objectives.
The Stages of Analytics Maturity
The AMM typically consists of several stages, each representing a higher level of analytical sophistication:
- Descriptive Analytics: This is the most basic level, focusing on what has happened in the past. It involves data collection and management, as well as basic reporting.
- Diagnostic Analytics: At this stage, organizations start to analyze data to understand why things happened. This involves more detailed data examination and correlation analysis.
- Predictive Analytics: Here, companies use statistical models and forecasts to predict future events based on historical data.
- Prescriptive Analytics: The most advanced stage, where analytics are used to recommend actions that can affect desired outcomes. This often involves complex simulations and optimization algorithms.
Each stage builds upon the previous one, requiring more sophisticated tools, technologies, and skillsets.
Technologies and Tools
As organizations move through the stages of the AMM, they typically adopt new technologies and tools to support their growing analytics needs. These may include:
- Data management platforms for organizing and storing large volumes of data.
- Business intelligence (BI) tools for reporting and visualization.
- Advanced statistical and machine learning software for predictive modeling.
- Optimization and simulation tools for prescriptive analytics.
Applications of Analytics Maturity Model
The AMM has applications across various industries and business functions, including:
- Marketing: For customer segmentation, targeting, and campaign optimization.
- Finance: For risk assessment, fraud detection, and financial forecasting.
- Operations: For supply chain optimization and process improvement.
- Human Resources: For workforce analytics and talent management.
Advancing Through the Maturity Model
To progress through the stages of the AMM, organizations must invest in the right people, processes, and technology. This involves:
- Developing or acquiring the necessary analytical skills and talent.
- Establishing clear processes for data governance and analytics.
- Investing in technology that supports advanced analytics capabilities.
Conclusion
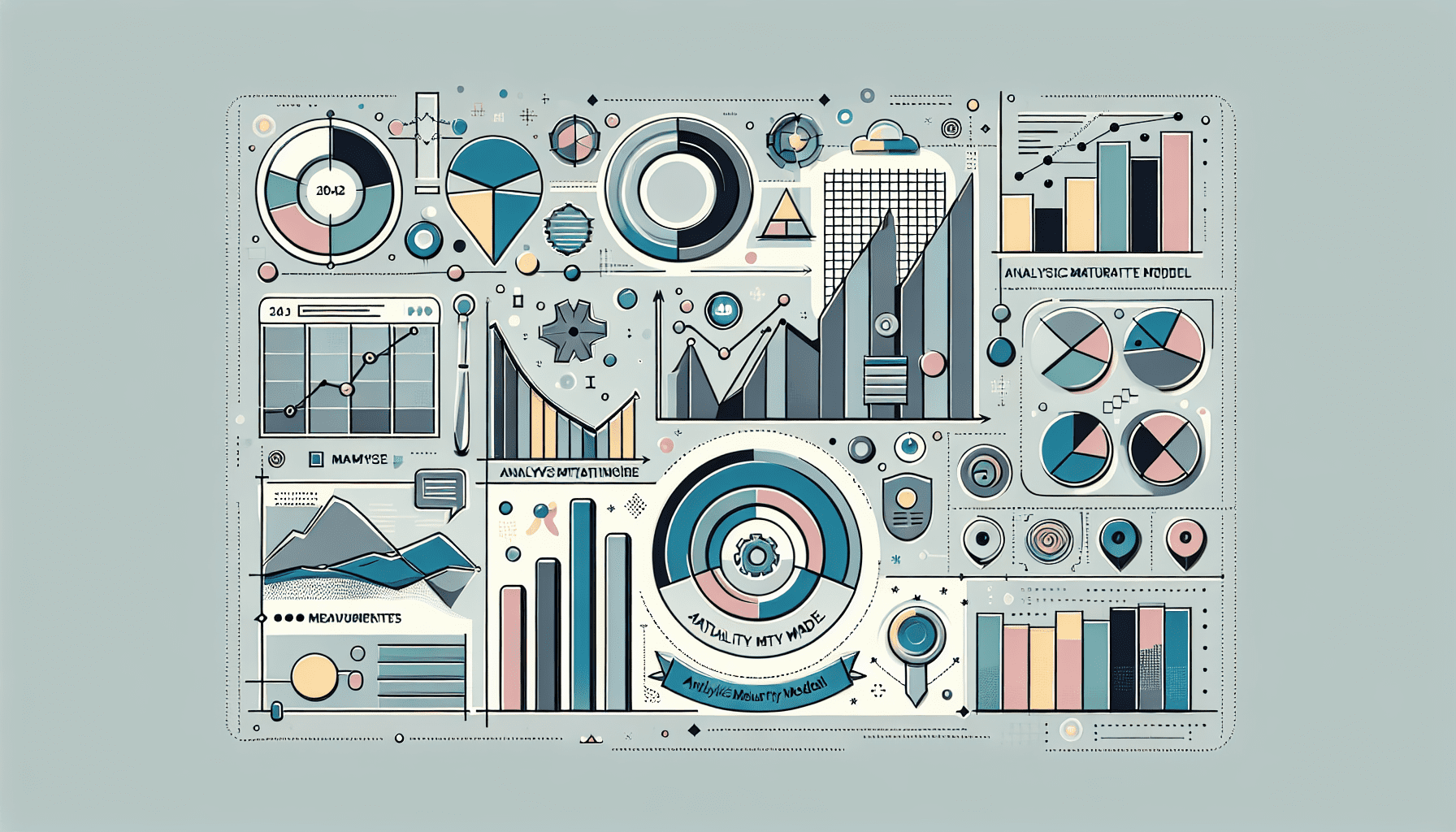
The Analytics Maturity Model is a critical tool for organizations seeking to leverage data for strategic advantage. By understanding where they stand on the maturity curve and what it takes to advance, companies can make informed decisions about their data and analytics investments.
The journey through the Analytics Maturity Model is not just about technology; it's a transformation that touches every aspect of the organization, from culture to operations to strategy.FAQs on Analytics Maturity Model
Q: Why is an Analytics Maturity Model important for organizations? A: An Analytics Maturity Model is important because it provides a structured approach for organizations to assess their current analytics capabilities, identify areas for improvement, and develop a strategic plan for advancing their use of data and analytics.
Q: Can small businesses benefit from an Analytics Maturity Model? A: Yes, businesses of all sizes can benefit from an Analytics Maturity Model. It helps small businesses to prioritize their analytics initiatives and focus on the most impactful areas.
Q: How often should an organization assess its analytics maturity? A: Organizations should regularly assess their analytics maturity to ensure they are keeping pace with technological advancements and evolving business needs. An annual assessment is common, but some may opt for more frequent reviews depending on their industry and rate of change.
Q: Who should be involved in the analytics maturity assessment process? A: A cross-functional team including business leaders, IT professionals, data scientists, and analytics experts should be involved in the assessment process to ensure a comprehensive understanding of the organization's analytics capabilities and needs.
Q: What are the challenges in advancing through the Analytics Maturity Model? A: Challenges include acquiring the right talent, establishing robust data governance, managing data quality, integrating disparate data sources, and aligning analytics initiatives with business goals. Resistance to change and a lack of understanding of the value of analytics can also be significant hurdles.
Sources
- Big data maturity model
- Analytics Maturity Model: Levels, Technologies, Applications
- What is Analytics Maturity Framework? - phData
- The 6 Stages of the Analytics Maturity Model - Graphable
- Analytics Maturity Model: The Journey toward Analytics Excellence
- Analytics Maturity Model -
- Analytics maturity model: how to get the most out of your data strategy
- Infographic: Understanding the Levels of Data Analytics Maturity
- Analytics Maturity Model Assessment: Benchmark Performance
- The Analytics Maturity Curve - Intel