Data Profiling A Comprehensive Guide to Understanding Your Data
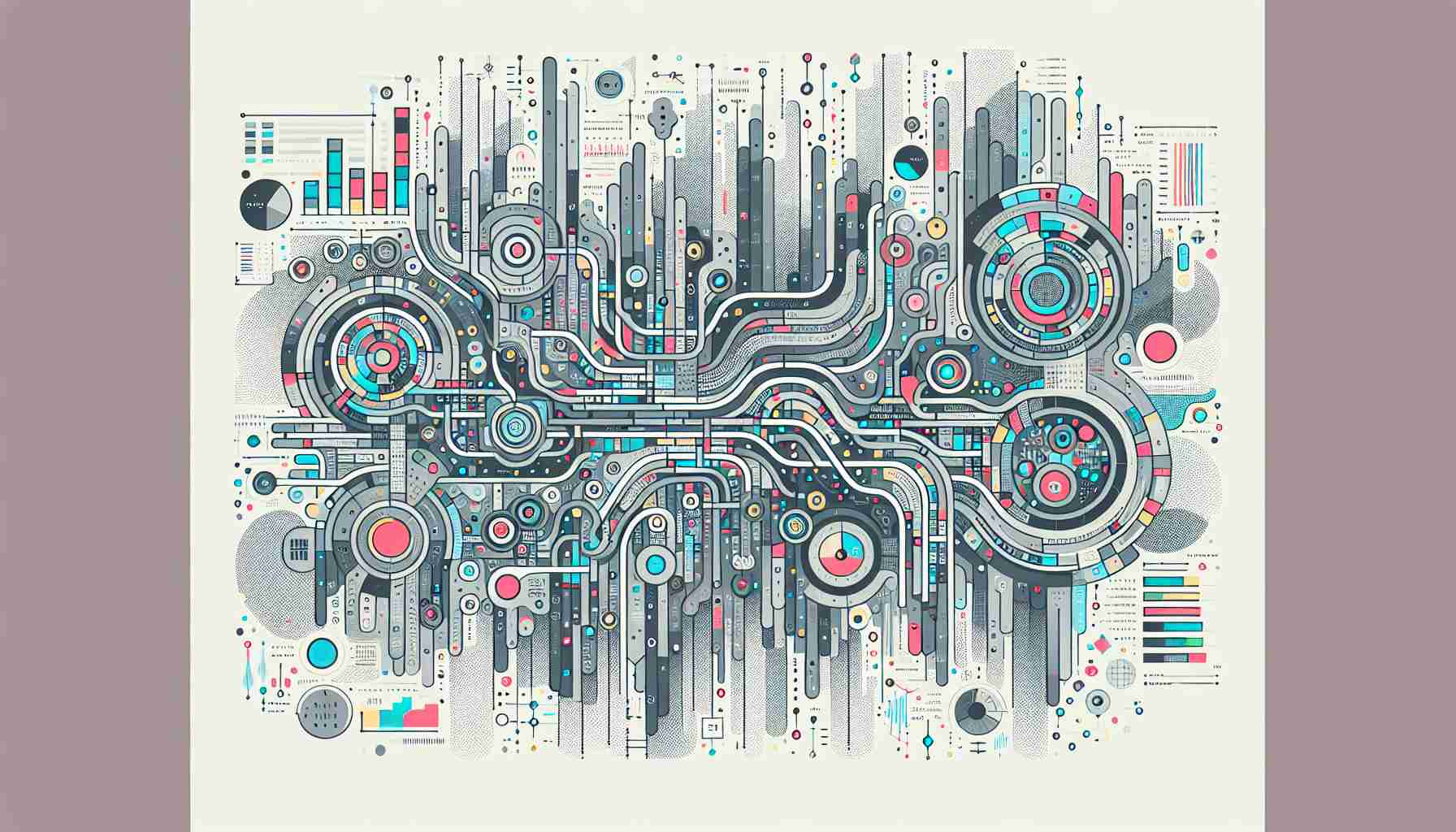
Data Profiling Briefly Summarized
- Data profiling is a critical process in data management that involves examining and understanding the structure, content, and interrelationships of data.
- It helps organizations assess data quality, identify potential for data projects, and ensure data conforms to standards or patterns.
- Through data profiling, businesses can improve searchability, tagging data with keywords or descriptions, and categorize it for better usage.
- The process is essential for risk assessment in data integration and for discovering metadata, which includes value patterns, key candidates, and functional dependencies.
- Data profiling enables an enterprise view of data, crucial for master data management and data governance, ultimately improving data quality and decision-making.
Data profiling is an indispensable step in the data analysis process, serving as the foundation for ensuring data quality and integrity. It is the meticulous examination of data available from various sources, aimed at extracting meaningful insights and summaries. This process not only reveals the nature of the data but also uncovers the underlying issues that could affect its usability and reliability.
Introduction to Data Profiling
At its core, data profiling is about gaining a deep understanding of data characteristics. It involves a series of activities that allow data analysts and engineers to make informed decisions about the handling and processing of data. The insights gained from data profiling can influence how data is stored, managed, and utilized across an organization.
The Importance of Data Profiling
Data profiling is not just a technical necessity; it is a business imperative. In an age where data drives decisions, the quality of data can be the difference between success and failure. By profiling data, organizations can:
- Ensure that data is suitable for its intended purpose.
- Enhance the accuracy and effectiveness of data analytics.
- Reduce the risk of errors when integrating new data sources.
- Facilitate better data governance and regulatory compliance.
The Data Profiling Process
The data profiling process can be broken down into several key steps:
- Data Collection: Gathering data from various sources, including databases, files, and external data streams.
- Data Analysis: Examining the structure, content, and relationships within the data to understand its format, consistency, and integrity.
- Metadata Discovery: Identifying and documenting metadata, such as data types, patterns, and value ranges.
- Data Quality Assessment: Evaluating the data for errors, inconsistencies, and deviations from expected standards or patterns.
- Reporting: Creating summaries and reports that provide insights into the data's quality and characteristics.
Techniques and Tools for Data Profiling
Data profiling can be performed using a variety of techniques and tools, ranging from simple spreadsheet applications to sophisticated data quality software. Some common methods include:
- Statistical Analysis: Using statistical measures to understand data distributions and identify outliers.
- Pattern Recognition: Detecting common patterns within data to understand its structure and consistency.
- Data Visualization: Employing graphical representations to identify trends, correlations, and anomalies.
- Automated Profiling Tools: Utilizing specialized software that can quickly profile large datasets and provide comprehensive reports.
Best Practices in Data Profiling
To achieve the best results from data profiling, organizations should adhere to several best practices:
- Start Early: Begin profiling data at the outset of any data project to identify issues before they become costly.
- Be Thorough: Profile all relevant aspects of data, including structure, content, and relationships.
- Iterate: Data profiling should be an ongoing process, with continuous refinement and re-evaluation as data evolves.
- Collaborate: Involve stakeholders from different departments to ensure a comprehensive understanding of data requirements and quality issues.
Conclusion
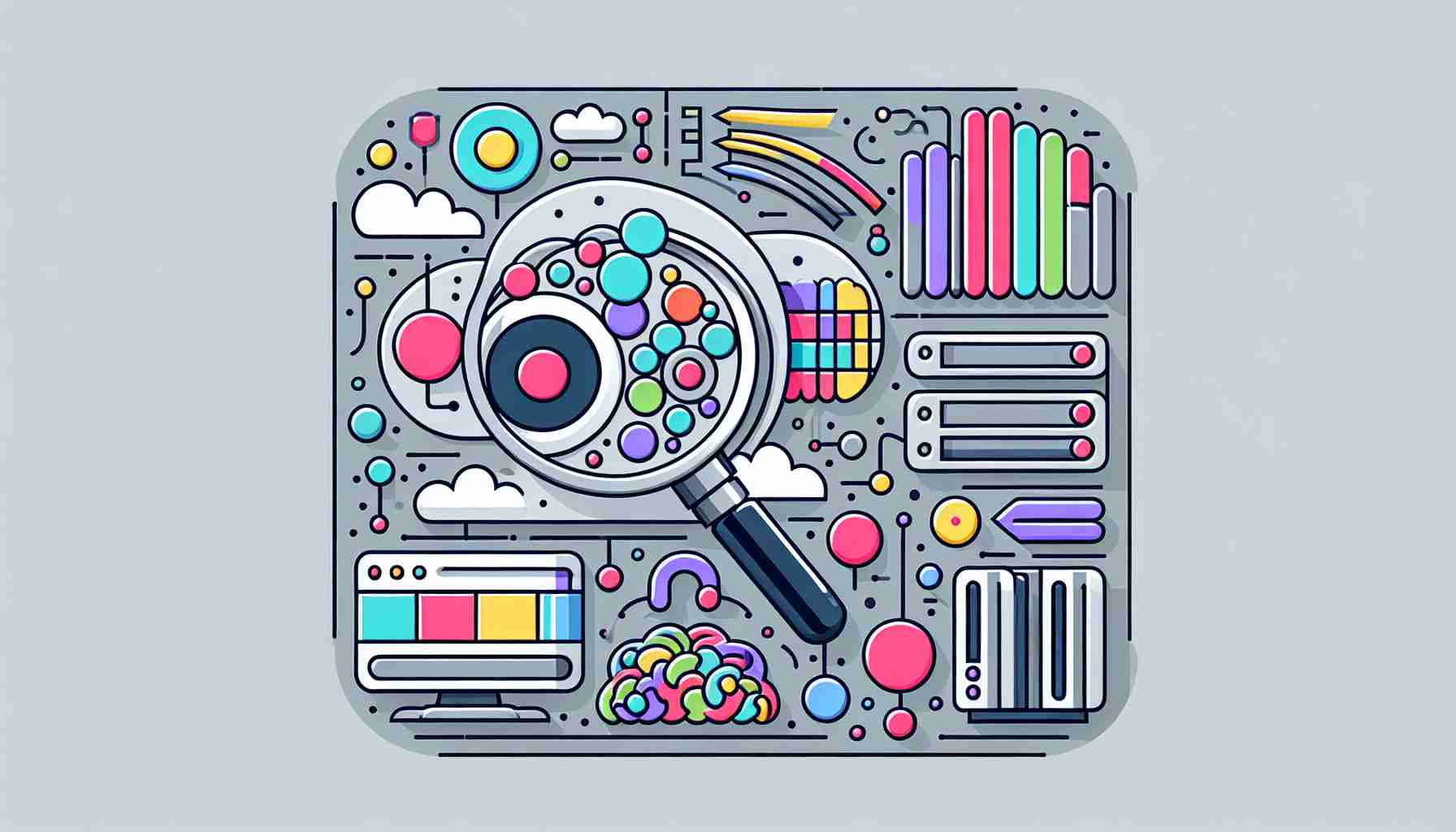
Data profiling is a vital activity that supports data-driven decision-making and enhances the overall quality of data. By thoroughly understanding their data, organizations can mitigate risks, improve operational efficiency, and gain a competitive edge. As data continues to grow in volume and complexity, the role of data profiling in managing this valuable asset becomes increasingly important.
FAQs on Data Profiling
What is data profiling? Data profiling is the process of examining, analyzing, and summarizing data to understand its structure, content, and interrelationships, and to assess its quality.
Why is data profiling important? Data profiling is important because it helps organizations ensure data quality, compliance, and readiness for use in various applications, including analytics and decision-making.
When should data profiling be done? Data profiling should be done early in the data lifecycle, before data is used for analysis or integrated into new systems, and should continue as an ongoing process to maintain data quality.
What tools are used for data profiling? There are many tools available for data profiling, ranging from simple database query tools to advanced data quality and analytics software designed specifically for profiling tasks.
How does data profiling improve decision-making? Data profiling improves decision-making by providing accurate and reliable data, which is essential for drawing valid conclusions and making informed business decisions.
Sources
- Data profiling
- What Is Data Profiling? Process, Best Practices and Tools - Panoply.io
- What is Data Profiling? - IBM
- What is data profiling and how does it make big data easier? | SAS
- What Is Data Profiling: Tools and Best Practices [2024]
- What is Data Profiling? - Definition from SearchDataManagement
- Data Profiling: What Is It & How Does It Drive Decision Making?
- Data profiling - Wikipedia
- Data Profiling: Definition, Techniques, Process & Examples - Atlan
- Definition of Data Profiling - Gartner Information Technology Glossary