Data Blending The Key to Comprehensive Data Analysis
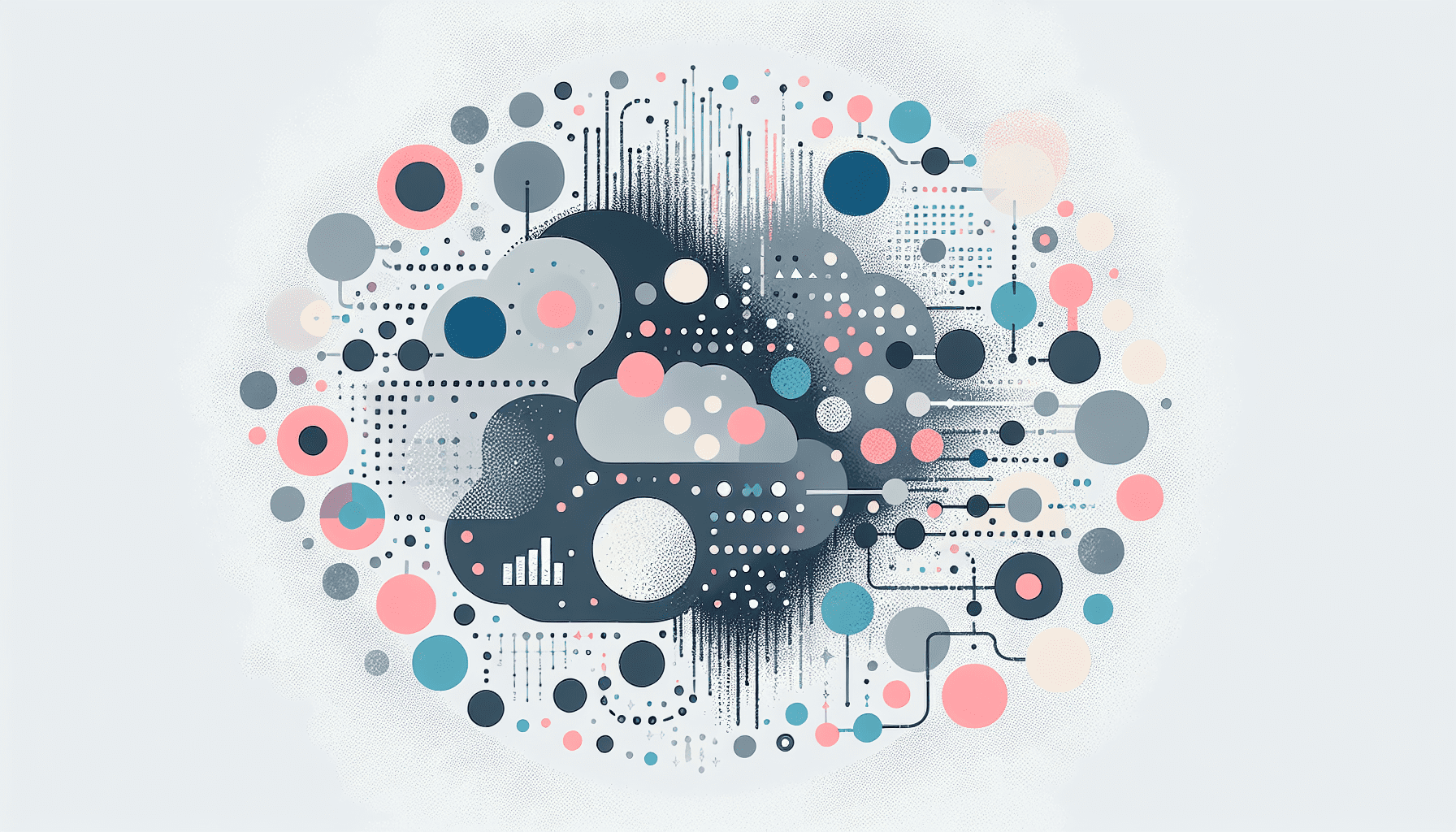
Data Blending Briefly Summarized
- Data blending is the process of combining data from multiple sources into a unified dataset.
- It enables business analysts to integrate big data quickly for timely and informed decision-making.
- Unlike traditional data integration, data blending is designed for speed and agility, often bypassing the need for extensive intervention by data scientists.
- This technique is crucial for performing deeper, more complex analyses that provide richer business intelligence.
- Data blending tools and platforms, such as Tableau, Alteryx, and Looker Studio, facilitate the merging of datasets with varying structures and formats.
Data blending has become an essential practice in the realm of data analysis, offering a pathway to unlock insights that are otherwise hidden within disparate data silos. As businesses continue to generate and collect vast amounts of data, the ability to efficiently merge and make sense of this information is critical. This article delves into the concept of data blending, its importance, methodologies, challenges, and best practices.
Introduction to Data Blending
In the modern data-driven business environment, organizations often find themselves managing a plethora of data sources, each containing valuable insights. Data blending is the act of combining this diverse data to create a cohesive dataset that can be analyzed to uncover deeper insights and inform strategic decisions.
Data blending is distinct from traditional data integration in that it focuses on the rapid merging of data sources to meet the immediate needs of data analysts. While data integration involves a more comprehensive and often time-consuming process of unifying data at a database level, data blending is performed at the dataset level, typically within an analytics tool.
Why Data Blending Matters
The significance of data blending lies in its ability to empower analysts to work with multiple data sources without the need for complex ETL (Extract, Transform, Load) processes or IT intervention. By quickly combining datasets, analysts can:
- Respond to business queries in real-time.
- Gain a holistic view of organizational data.
- Identify correlations and trends across different data sets.
- Enhance the quality of business intelligence and reporting.
How Data Blending Works
Data blending typically involves several key steps:
- Identifying Data Sources: Analysts select the primary and secondary data sources that will be blended.
- Data Extraction: Data is extracted from the chosen sources, which may include databases, spreadsheets, cloud storage, or other repositories.
- Data Cleansing: The extracted data is cleaned to ensure accuracy and consistency, which may involve removing duplicates, correcting errors, and standardizing formats.
- Data Merging: The clean datasets are combined using common fields or keys. This might be a straightforward process or require complex transformations.
- Data Aggregation: The blended data is often aggregated to summarize information and facilitate analysis.
- Analysis and Visualization: The final blended dataset is used for analysis, with results often visualized in dashboards or reports.
Tools and Technologies for Data Blending
Several tools and technologies have emerged to support data blending, with some of the most prominent being:
- Tableau: Known for its data visualization capabilities, Tableau also offers robust data blending features that allow users to combine multiple data sources on a single worksheet.
- Alteryx: Alteryx provides a platform for data blending that is designed for analysts to perform complex data combinations without coding.
- Looker Studio (formerly Google Data Studio): Looker Studio enables users to blend data from different sources, such as BigQuery and spreadsheets, to create comprehensive reports and dashboards.
Challenges in Data Blending
While data blending offers numerous benefits, it also presents challenges:
- Data Quality: Ensuring the accuracy and consistency of data from multiple sources can be difficult.
- Data Governance: Blending data from various sources can raise concerns about data security, privacy, and compliance.
- Performance: Blending large volumes of data can be resource-intensive and may impact system performance.
Best Practices for Data Blending
To overcome these challenges and ensure successful data blending, consider the following best practices:
- Establish clear data governance policies to maintain data security and compliance.
- Prioritize data quality by implementing robust data cleansing and validation processes.
- Optimize performance by blending data at the right level of detail and using efficient tools.
Conclusion
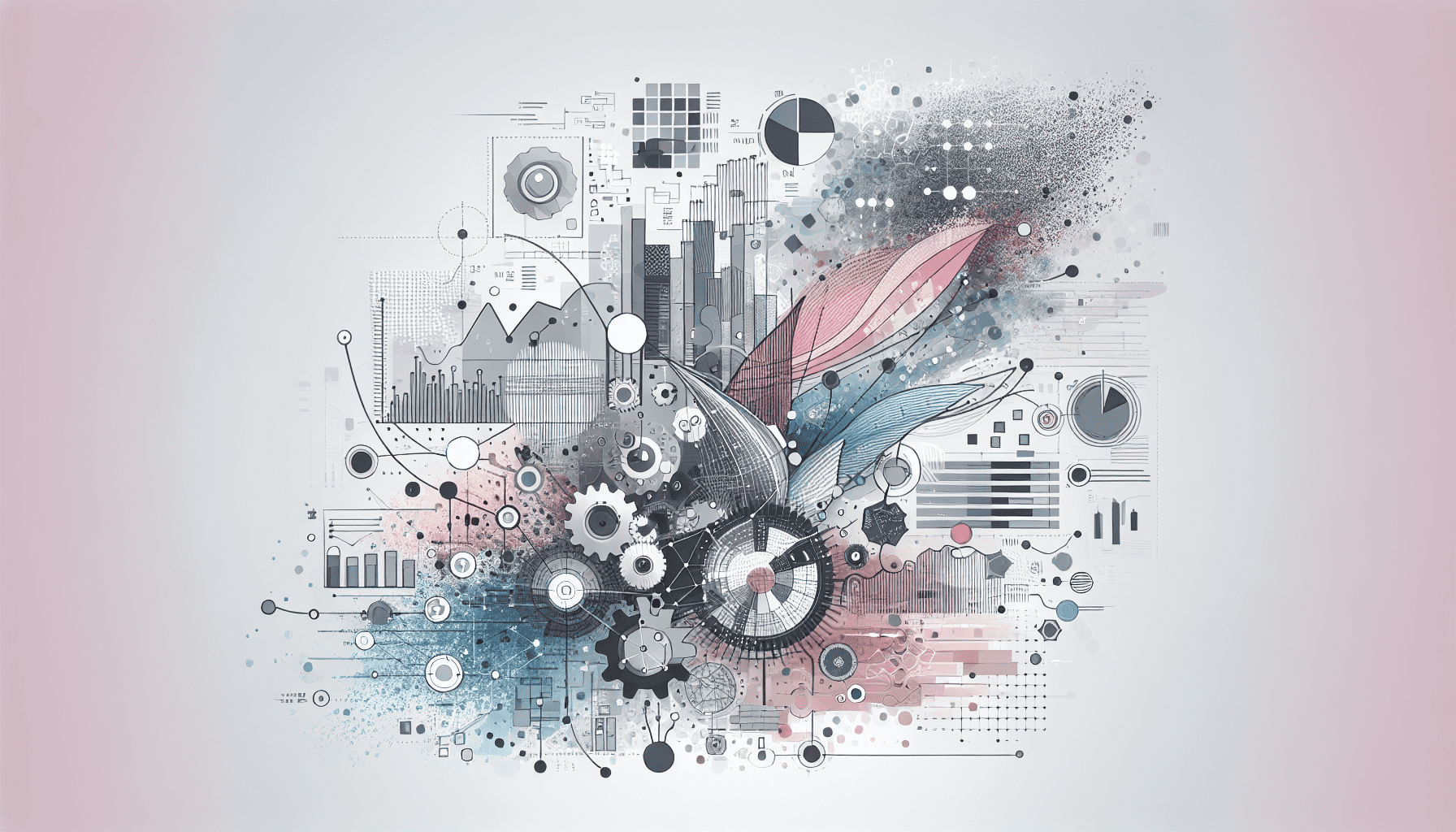
Data blending is a powerful technique that enables analysts to quickly combine data from various sources to gain comprehensive insights. By leveraging the right tools and adhering to best practices, organizations can harness the full potential of their data to drive better business outcomes.
FAQs on Data Blending
What is the difference between data blending and data integration? Data blending is a rapid method for combining data at the dataset level for immediate analysis, while data integration is a more comprehensive process of unifying data at the database level.
Can data blending be automated? Yes, many data blending tools offer automation features to streamline the process, reducing manual effort and the potential for errors.
Is data blending suitable for all types of data? Data blending can be used with various types of data, but it is essential to ensure that the data is compatible and that the blending process preserves data integrity.
Sources
- Data blending
- Blend Your Data - Tableau Help
- Data blending - Wikipedia
- What Is Data Blending & Why Is It Important? - Alteryx
- How blends work - Looker Studio Help
- What is Data Blending? - Sisense
- Blend on Summary Data - Tableau Help
- What is Data Blending in Tableau And How to Implement It
- What is Data Blending in Tableau? - Softcrylic
- Why you should blend instead of join in Tableau - West Monroe
- Tableau - Data Blending - Tutorialspoint