Real-Time Analytics The Power of Immediate Insights
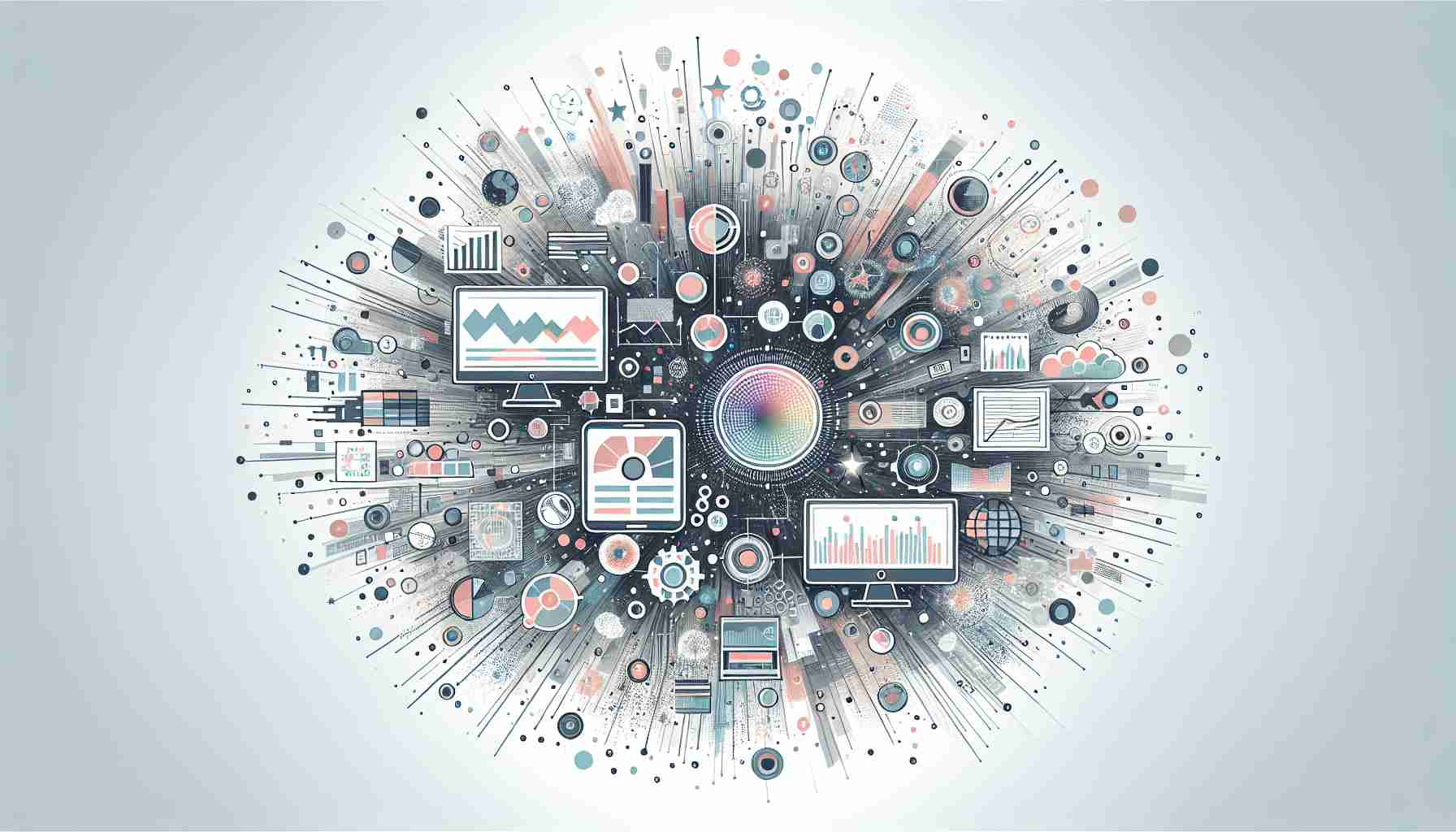
Real-Time Analytics Briefly Summarized
- Real-time analytics involves the immediate processing and analysis of data as it is captured.
- It enables businesses to respond swiftly to changing conditions, detect patterns, and capitalize on opportunities.
- The core requirements are access to fresh data and the ability to perform fast queries.
- Real-time analytics is distinguished from batch processing by its instantaneous nature, providing actionable insights without delay.
- With the advent of technologies like Google Analytics 4 and BigQuery, real-time analytics is becoming more integrated into cloud services and accessible to a wider range of users.
Real-time analytics is a transformative approach to data analysis that allows organizations to make decisions based on the most current information available. In a world where data is generated at an unprecedented rate, the ability to analyze and act upon data in real time is a significant competitive advantage. This article delves into the intricacies of real-time analytics, exploring its importance, the technology that enables it, and its various applications across industries.
Introduction to Real-Time Analytics
In the realm of data analysis, the term "real-time" refers to the processing and analysis of data as soon as it is generated or received. Unlike traditional batch processing, where data is collected over a period and analyzed in intervals, real-time analytics strives to minimize the time between data creation and actionable insights.
The essence of real-time analytics lies in its ability to provide immediate feedback, allowing businesses to react promptly to emerging trends, operational issues, or customer behaviors. This immediacy is crucial in environments where conditions change rapidly, such as financial markets, online retail, and social media platforms.
The Technology Behind Real-Time Analytics
Real-time analytics is made possible by a combination of advanced technologies and architectural choices. At its core, the system must be capable of handling high-velocity data streams and performing rapid queries. This is achieved through:
- In-Memory Computing: Storing data in RAM, as opposed to slower disk-based storage, for faster access and processing.
- Stream Processing: Analyzing data on the fly as it streams into the system, rather than storing it first and then processing.
- Distributed Architectures: Utilizing a network of computers to process data in parallel, enhancing speed and scalability.
- Complex Event Processing (CEP): Identifying patterns and relationships across multiple streams of data in real time.
Applications of Real-Time Analytics
Real-time analytics has a wide array of applications across various sectors:
- E-commerce: Personalizing customer experiences by recommending products based on real-time browsing behavior.
- Finance: Monitoring stock market fluctuations to make immediate trading decisions.
- Healthcare: Tracking patient vitals in critical care for timely intervention.
- Manufacturing: Detecting anomalies in production lines to prevent equipment failure.
- Transportation: Optimizing routes for delivery vehicles based on current traffic conditions.
Real-Time Analytics in Action
One of the most prominent examples of real-time analytics in action is Google Analytics. As a web analytics service, it allows website owners to track and report traffic in real time. With the introduction of Google Analytics 4 (GA4), users have access to more integrated features, such as a natural connection with Google's BigQuery. This integration signifies a push towards a more comprehensive cloud analytics offering, where real-time data can be analyzed alongside historical data for deeper insights.
The Future of Real-Time Analytics
The future of real-time analytics is closely tied to advancements in artificial intelligence (AI) and machine learning (ML). As these technologies evolve, they will enhance the predictive capabilities of real-time systems, enabling even more nuanced and anticipatory responses to data.
Conclusion
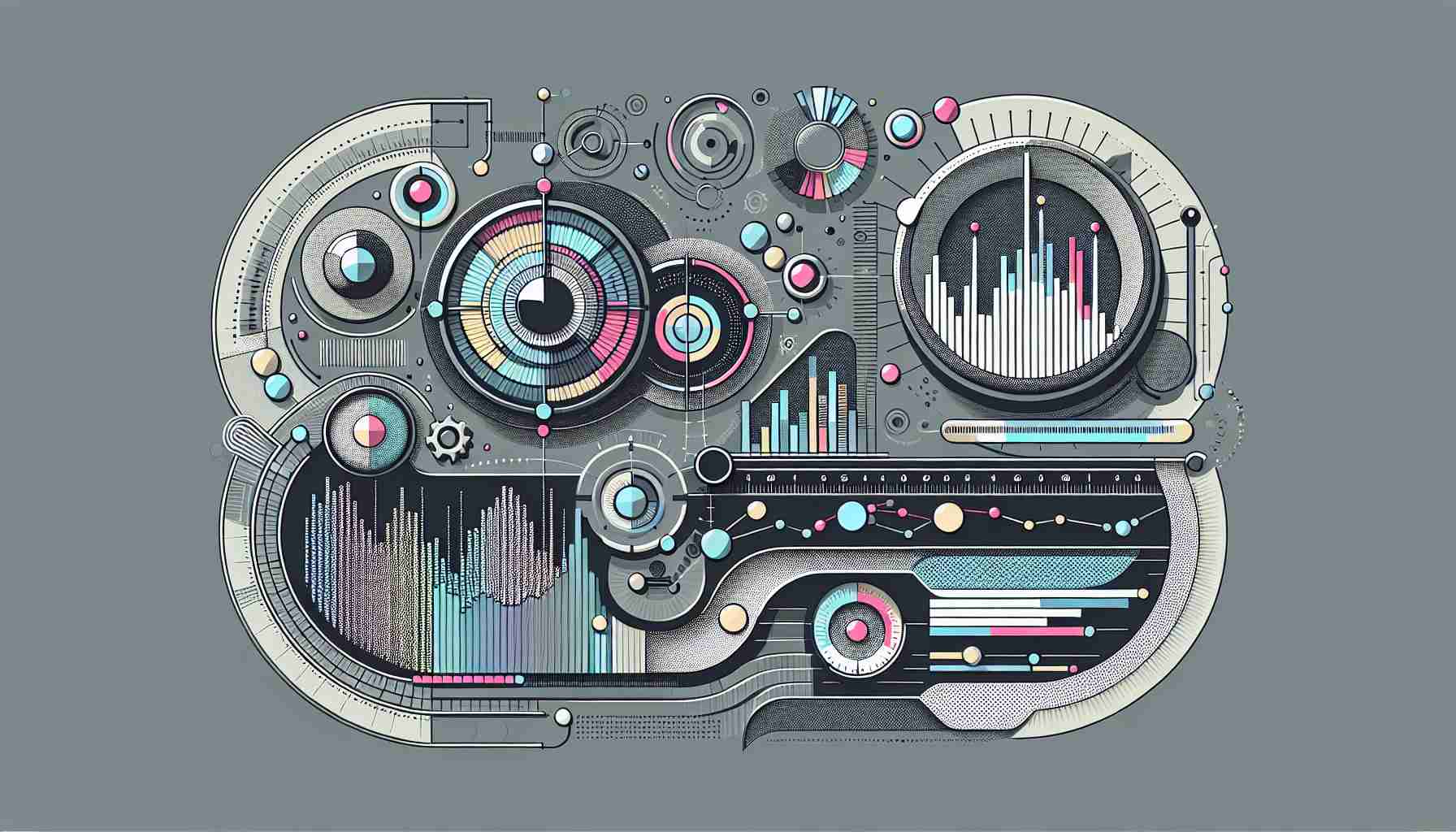
Real-time analytics represents a paradigm shift in how businesses approach data. By enabling immediate insights and responses, organizations can stay ahead of the curve, adapt to changes swiftly, and deliver enhanced customer experiences. As technology continues to advance, the scope and impact of real-time analytics will only grow, solidifying its role as a critical tool in the data-driven decision-making process.
FAQs on Real-Time Analytics
-
What is the difference between real-time analytics and batch processing? Real-time analytics processes data instantly as it arrives, while batch processing involves collecting data over a period and analyzing it in intervals.
-
How does real-time analytics impact decision-making? It allows businesses to make informed decisions based on the latest data, leading to more timely and effective actions.
-
What industries benefit from real-time analytics? Numerous sectors, including finance, e-commerce, healthcare, manufacturing, and transportation, benefit from the immediacy of real-time analytics.
-
What are the challenges of implementing real-time analytics? Challenges include managing high-velocity data streams, ensuring low-latency processing, and integrating real-time analytics into existing IT infrastructures.
-
How does AI and ML influence real-time analytics? AI and ML enhance the predictive power of real-time analytics, allowing for more advanced and anticipatory data analysis.
Sources
- Google Analytics
- What is real-time analytics? - TechTarget
- What is Real Time Analytics? - Sisense
- Real-Time Analytics Explained - Rockset
- Real-Time Analytics With Examples - MongoDB
- What Is Real-Time Analytics? | StarTree
- What is Real Time Analytics? Definition and FAQs | HEAVY.AI
- What are Real-Time Analytics: Examples & Benefits - SolveXia
- Overview of Real-Time Analytics - Microsoft Fabric
- Real-Time Analytics Overview, Technology, and Use Cases - Intel